Agentic Value Creation
Note: This is an exploration of emerging trends in agentic AI
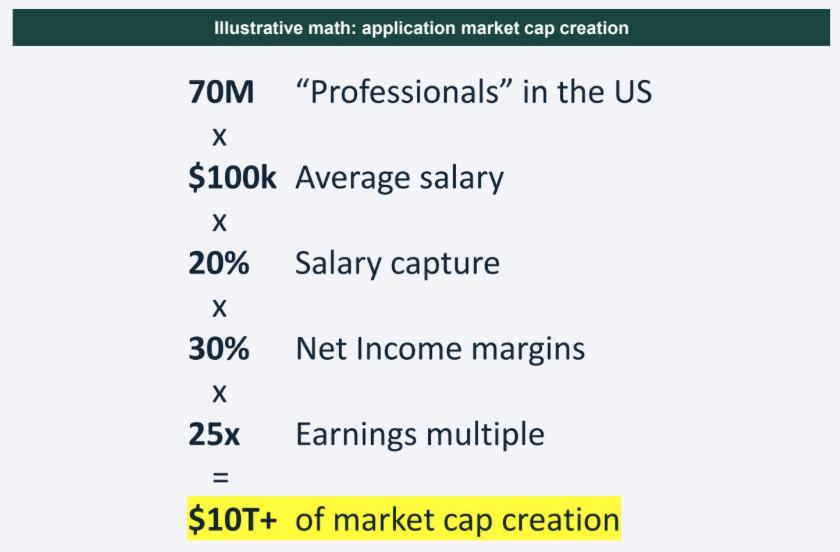
The numbers are staggering, even by Big Tech standards. An estimate of app-level market cap creation in the US alone amounts to $10tn+ — roughly equivalent to the combined market caps of Apple, Microsoft, and Google at their peaks. This unprecedented potential has sparked what could be the largest concentrated R&D investment in history: Big Tech companies are projected to spend $320bn on AI development in 2025, up from $230bn in 2024 (a ~40% increase).
These investment levels might seem excessive until you consider the stakes. Dario Amodei (CEO, Anthropic) has gone on record to say that we could see powerful AI akin to AGI as early as 2026, with Sam Altman (CEO, OpenAI) suggesting that “AGI will probably get developed during this president’s term”.
Before we reach AGI, however, we’re seeing the emergence of increasingly capable AI agents — systems that can operate autonomously for extended periods, handling complex tasks that previously required human expertise. Devin, for instance, represents an early glimpse of this capability. OpenAI frames this evolution through distinct stages, where “Agents” refers to AI systems that can spend several days taking actions on a user’s behalf:”
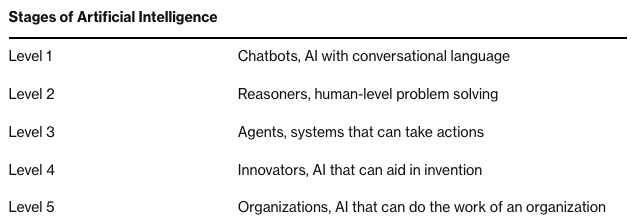
Anthropic, on the other hand, categorizes agents as systems where LLMs dynamically direct their own processes and tool usage, maintaining control over how they accomplish tasks. This definition highlights a key aspect of agent development: the progression from simple task execution to autonomous decision-making. OpenAI’s latest framework captures this evolution, as Srinivas Narayanan, VP of Engineering at OpenAI, outlined in a recent talk:
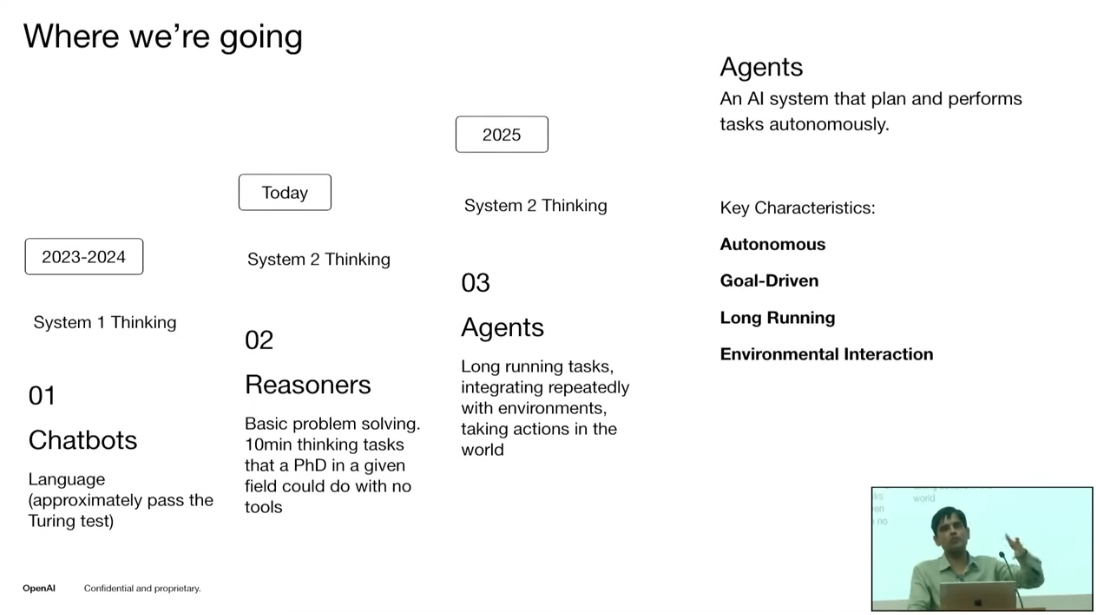
The advancement from Level 1 (basic chatbots) to Level 2 (reasoning systems) in just one year signals an inflection point in AI development, creating opportunities not just for companies building foundational models, but for those operating across the entire AI value chain. We’re already seeing evidence of this value creation: Accenture drove $3bn in revenue from just GenAI services in 2024 while OpenAI expects $3.7bn revenue in the same period.
To understand where this unprecedented value creation materializes across the AI stack, let’s examine the complete value chain:
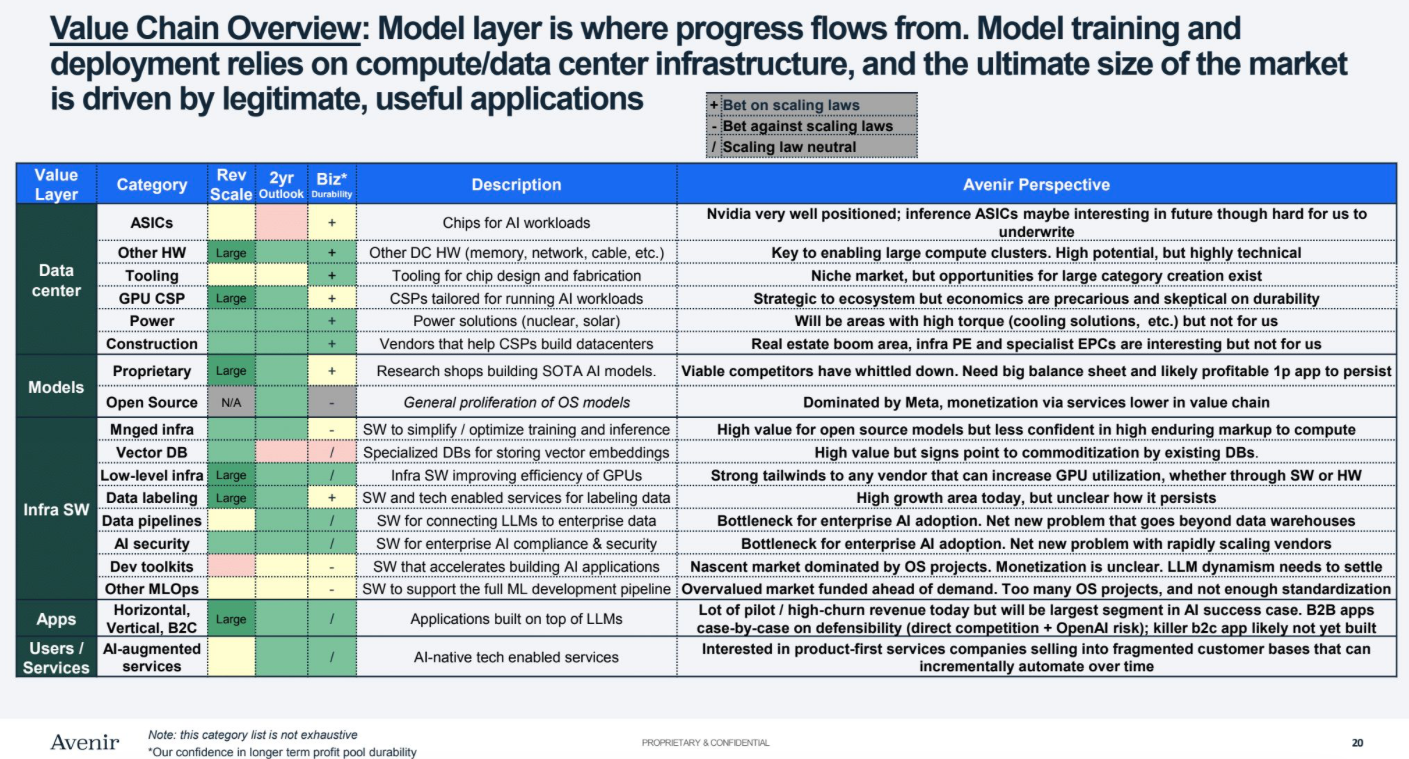
The ultimate market size is driven by legitimate, useful applications across this stack. Particularly noteworthy is the emphasis on infrastructure software and enterprise AI adoption as key bottlenecks that need to be solved for the market to reach its full potential.
As an example, Agentic AI platforms represent a unique opportunity here, spanning multiple layers of the value chain - from apps to infrastructure software to models. Salesforce’s recently launched Agentforce demonstrates this cross-layer integration in action. According to Marc Benioff, the platform’s initial traction has been remarkable:
… And you also know that in the last week of the quarter, Agentforce went production. We delivered 200 deals, and our pipeline is incredible for future transactions. We can talk about that with you on the call, but we’ve never seen anything like it. We don’t know how to characterize it. This is really a moment where productivity is no longer tied to workforce growth, but through this intelligent technology that can be scaled without limits.
The impact extends beyond enterprise solutions. In the consumer space, Microsoft’s vision points to a fundamental shift in how we interact with AI agents. Satya Nadella articulates this in a recent interview with Dwarkesh:
Dwarkesh Patel
You mentioned briefly what your own workflow—how your own workflow has changed as a result of AIs. I’m curious if we can add more color to what will it be like to run a big company when you have these AI agents that are getting smarter and smarter over time?
Satya Nadella
It’s interesting you ask that. I was thinking, for example, today if I look at it, we are very email heavy. I get in in the morning, and I’m like, man my inbox is full, and I’m responding, and so I can’t wait for some of these Copilot agents to automatically populate my drafts so that I can start reviewing and sending.
But I already have in Copilot at least ten agents, which I query them different things for different tasks. I feel like there’s a new inbox that’s going to get created, which is my millions of agents that I’m working with will have to invoke some exceptions to me, notifications to me, ask for instructions.
That’s why I think of this Copilot, as the UI for AI, is a big, big deal. So basically, think of it as: there is knowledge work, and there’s a knowledge worker. The knowledge work may be done by many, many agents, but you still have a knowledge worker who is dealing with all the knowledge workers. And that, I think, is the interface that one has to build.
This vision of ubiquitous agents raises a critical challenge: while we do see empirically that the cost to use a given level of AI falls about 10x every 12 months, unchecked agent spawning could sharply inflate enterprise costs. This is where persistent agent frameworks become essential - systems that maintain continuity and efficiency in AI agent operations across multiple tasks and timeframes. Unlike traditional AI implementations that spawn new instances for each task, these frameworks optimize resource usage and maintain context across operations. As Barry Zhang (Applied AI, Anthropic) notes in a recent chat hosted by Anthropic:
One thing I’ve been really impressed by is just, I work with some really resourceful startups and they can do everything within one LLM call and the orchestration around the code, which will persist even as the model gets better, is kind of their niche. And I always get very happy when I see one of those because I think they can reap the benefit of future capability improvements.
But several critical challenges outside of costs emerge as we progress toward this vision:
How do we maintain goal alignment across chains of autonomous agents?
This question of control and emergence in complex systems shares similarities with Conway’s Game of Life. The game, devised by mathematician John Conway in 1970, is a cellular automaton that simulates complex systems through simple rules. Played on an infinite grid of cells (each “alive” or “dead”), it evolves autonomously: cells survive with 2-3 neighbors, die with fewer or more, and new cells birth with exactly 3 neighbors. This zero-player game generates intricate patterns — gliders, oscillators, and self-replicating structures, illustrating how simple rules can yield complex, unpredictable behavior.
Alex illustrates this challenge through a thought experiment:
Suppose that we are working with an instance of Life with a very large grid, say 1030 rows by 1030 columns. Now suppose that I give you control of the initial on/off configuration of a region of size 1020 by 1020 in the top-left corner of this grid, and set you the goal of configuring things in that region so that after, say, 1060 time steps the state of the whole grid will resemble, as closely as possible, a giant smiley face.
![]()
The cells outside the top-left corner will be initialized at random, and you do not get to see what their initial configuration is when you decide on the initial configuration for the top-left corner.
The control question is: Can this goal be accomplished?
This mirrors a crucial question in agentic AI: can we initialize a “master” agent that effectively coordinates multiple subordinate agents toward specific business outcomes, even amid uncertainty? The challenge becomes especially acute when outcomes lag actions by months or years - how do we ensure agent actions remain aligned with intended goals over time? There’s a real risk that unconstrained agentic systems could evolve in unexpected, potentially “cancerous” ways, optimizing for the wrong metrics or creating unintended consequences.
When does the “master” AI agent know when to stop?
Nature offers an interesting parallel to this control challenge in the process of morphogenesis. As cells self-organize to build complex organisms, each cell somehow “knows” its role and when to stop growing. Despite understanding many of the genes involved in regeneration, we still haven’t decoded the fundamental algorithm that tells cells how to build and remodel organs to reach precise anatomical goals. This biological orchestration problem, which you can explore in this interactive model, mirrors the challenges we face in managing autonomous AI agents.
The very capabilities that make AI agents powerful - autonomy, persistence, and self-improvement - also make them challenging to control and direct. The winners in this space will likely be those who can build frameworks that balance autonomy with accountability. The path forward will likely be iterative and relatively slow compared to the pace we’re used to. In the immediate future (2025-2026), we’ll likely see relatively constrained implementations - AI agents operating within clear boundaries, with human knowledge workers serving as active orchestrators rather than passive observers. The “master” agent may start as more of an intelligent dashboard than a true autonomous controller. But as our understanding of multi-agent systems evolves, we might discover more robust patterns for self-regulating agent networks.
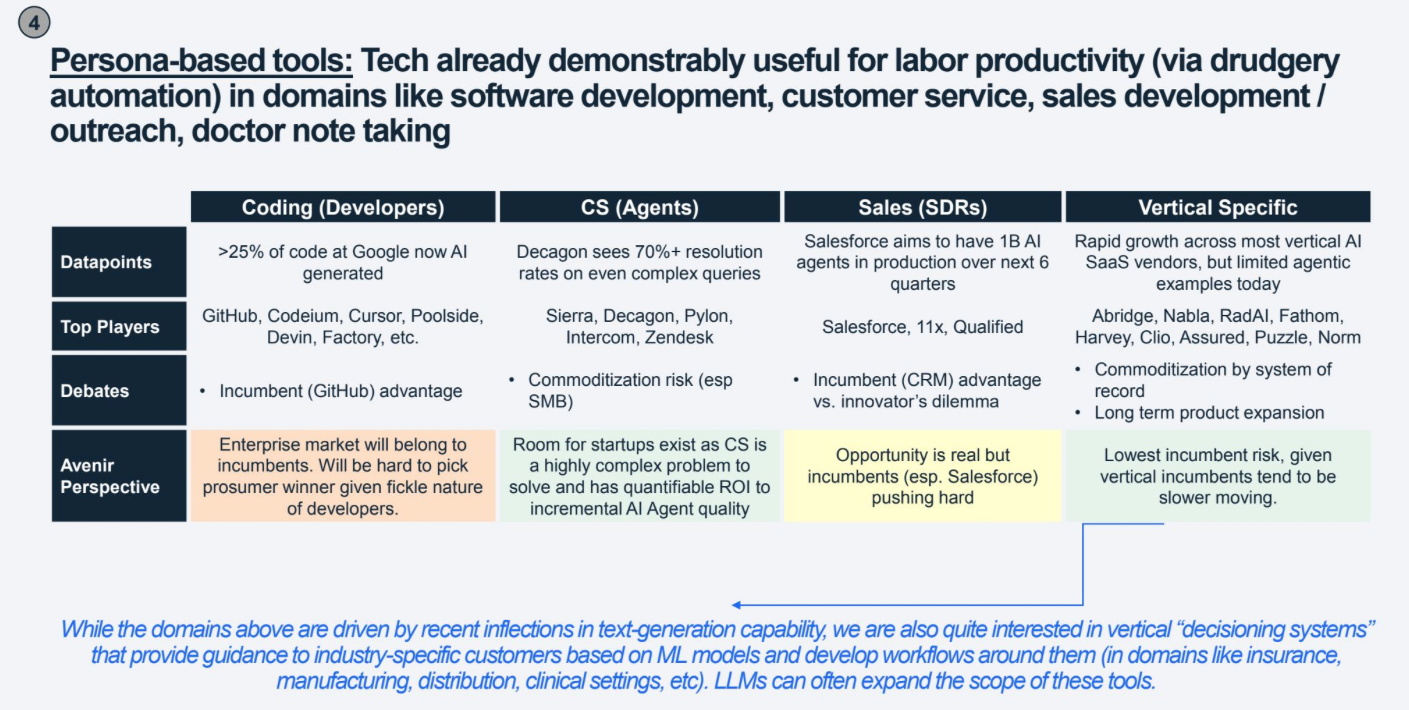
The $10tn opportunity will likely unfold through vertical-first expansion followed by horizontal consolidation, with the 2025-2026 window will likely be dominated by vertical solutions, with horizontal orchestration platforms emerging as winners validate their approaches across multiple industries.
Update (25th Feb): Anthropic just announced Claude 3.7 Sonnet and Claude Code. More importantly, Anthropic has adjusted its timeline, now projecting Claude to be “innovation-ready” by 2027 — suggesting AGI timelines may be extending slightly beyond previous projections. Maybe OpenAI clinches the title sooner, but OpenAI’s path forward appears more challenging, with Microsoft reportedly canceling data center leases. Time will tell.
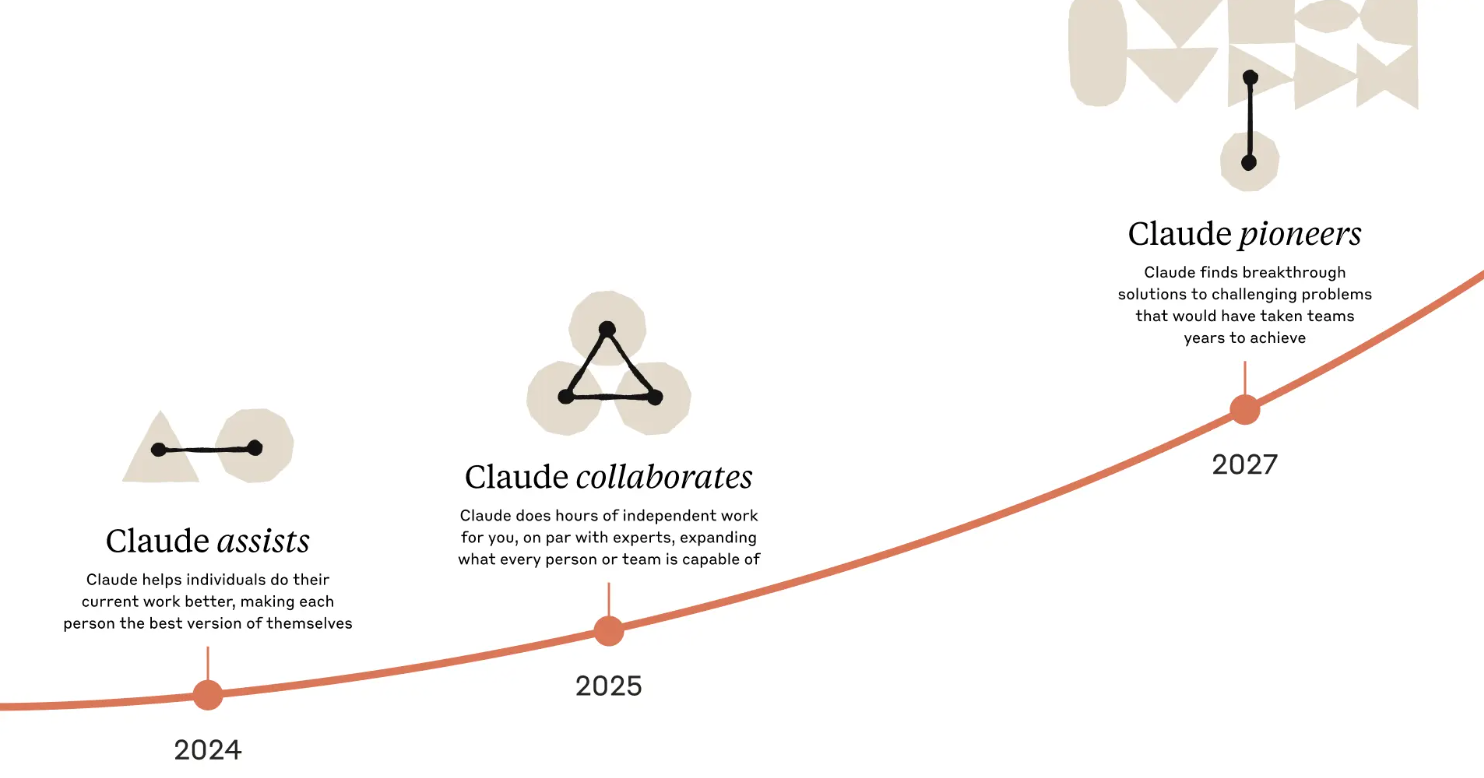