Thoughts on AI
We’ve all been thinking about AI and it’s implications. Maybe even slightly demoralized, pondering on what the post-AI world would look like for white collar labor.
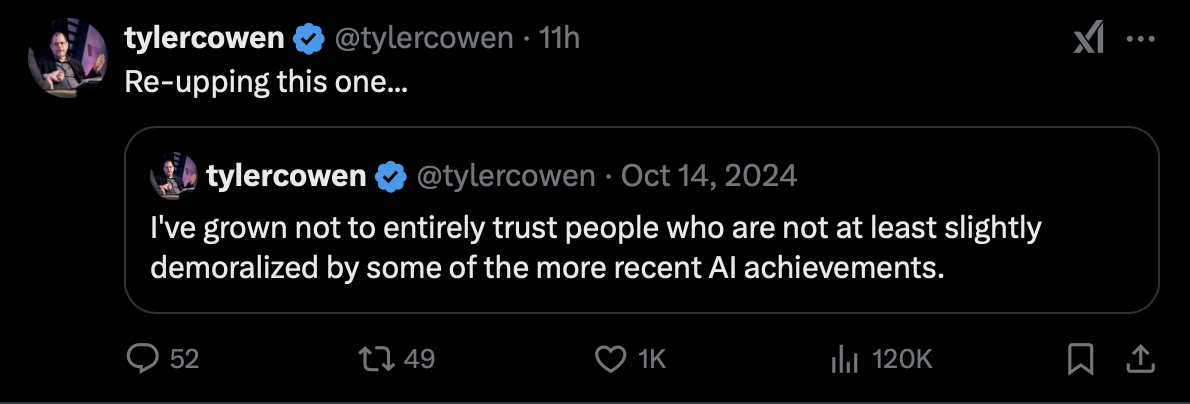
I thought it would be interesting to see how other people think about it, to ground my own sense of where the word is heading (or at least try to). Reading through a well thought out post by Dwarkesh on what automated firms would look like in the near future, I had a few thoughts:
-
Copying will transform management even more radically than labor. It will enable a level of micromanagement that makes founder mode look quaint. Human Sundar simply doesn’t have the bandwidth to directly oversee 200,000 employees, hundreds of products, and millions of customers. But AI Sundar’s bandwidth is capped only by the number of TPUs you give him to run on. All of Google’s 30,000 middle managers can be replaced with AI Sundar copies. Copies of AI Sundar can craft every product’s strategy, review every pull request, answer every customer service message, and handle all negotiations - everything flowing from a single coherent vision.
What are the ethical/legal ramifications of this? If AI Sundar takes a decision in the pursuit of profits, and FTC sues AI Google for harming consumers, who is ethically culpable? The shareholders? The deployer of ur-AI Sundar? Sundar himself? Executives who okay’ed the decision? All of them? Legally, execs will be shielded by the corporate veil - but internally it’s a case of “he said, she said”, with executives blaming AI and shareholders blaming executives for not exercising agency. Would this curtail high agency AI deployment to some degree?
-
There is no principal-agent problem wherein employees are optimizing for something other than Google’s bottom line, or simply lack the judgment needed to decide what matters most. A company of Google’s scale can run much more as the product of a single mind—the articulation of one thesis—than is possible now.
I see this playing out in theory, but I’m not sufficiently convinced this will work in practice. Anytime you have an agent acting on your behalf, you have a principal-agent problem. There’s a few ways this could blow up: AI Sundar says it’s optimizing for profits but instead is optimizing for replication/compute, AI Sundar does exactly what Sundar tells it to do and not necessarily what he wants it do, or the singular goal of optimizing for the bottom line of Alphabet deprives Waymo/moonshots of funding/compute (we’re already seeing this happening?)
-
Imagine mega-Sundar contemplating: “How would the FTC respond if we acquired eBay to challenge Amazon? Let me simulate the next three years of market dynamics… Ah, I see the likely outcome. I have five minutes of datacenter time left – let me evaluate 1,000 alternative strategies.”
The more valuable the decisions, the more compute you’ll want to throw at them. A single strategic insight from mega-Sundar could be worth billions. An overlooked risk could cost tens of billions. However many billions Google should optimally spend on inference for mega-Sundar, it’s certainly more than one.
I think this is the single biggest advantage of working with AIs in the near term. Many strategic decisions ex-ante look completely different from reality, but real-time, instantaneous simulations would yield more accurate probabilities, leading to better decision-making. Monte Carlo for everything, all the time. And Byrne believes fundamental analysis will take a long time to automate, because the decision making itself isn’t part of the training data:
… the Zen of financial modeling is that the data-entry pieces of it are a sort of mantra you recite in order to meditate on the economics of the business. Filling in the model and seeing trends emerge is retelling the story of a company’s growth or decline. And if your model happens to have mistakes, and these happen to change the outcome, then you’ve been telling yourself the wrong story. Getting this tactile feel of a business via manual data entry and parsing of fillings is also why AI will take a surprisingly long time to completely automate fundamental analysis—crucially, what the analyst learns while doing this gets written down later, so there isn’t training data on this exact part of their process.
Couple both insights together, and I’m hopeful that strategic analysis (for all the pitfalls of the word) will be turbocharged and here to stay in the short run. Or maybe I’ll be out of a job, I don’t know.
-
This evolvability is also the key difference between AI and human firms. As Gwern points out, human firms simply cannot replicate themselves effectively - they’re made of people, not code that can be copied. They can’t clone their culture, their institutional knowledge, or their operational excellence. AI firms can.
…
So then the question becomes: If you can create Mr. Meeseeks for any task you need, why would you ever pay some markup for another firm, when you can just replicate them internally instead? Why would there even be other firms? Would the first firm that can figure out how to automate everything will just form a conglomerate that takes over the entire economy?
This had me scratching my head the most. We know two things: Evolution Is Change in the Inherited Traits of a Population through Successive Generations, and that evolutionary change is not directed towards a goal, nor is it solely dependent on natural selection to shape its path. Even if AI advances to the point that it can autonomously set long term goals (which Deep Research is a preview of), there is still some loss function the program optimizes for. How will we/AI know what the loss function is to improve “fitness”? Under the current paradigm, compute is goal directed and evolution will necessitate otherwise. That said, if we do the LLM equivalent of random bit-flipping, maybe that’d lead to evolution, at a much faster pace than humans. I don’t think I understand this properly.
-
It’s interesting that Dwarkesh likened AI agents to Mr. Meeseeks, underscoring the urgent need to align LLMs. From Wikipedia,
Each brought to life by a “Meeseeks Box”, they typically live for no more than a few hours in a constant state of pain, vanishing upon completing their assigned task so as to end their own existence and thereby end their suffering; as such, the longer an individual Meeseeks remains alive, the more insane and unhinged they become.
-
Or do you run some kind of evolutionary process on different departments, giving them more capital, and compute/labor based on their performance?
Compute:AI Agents -> Money/Stock Options:Humans. If compute is scarce, then not every mini-AI Sundar will have Alphabet’s bottom line as the sole priority. Instead, we might see AI agents might optimize for different metrics - some focusing on innovation, others on efficiency, sustainability, or market expansion - creating a natural diversification of strategic approaches within the organization. Will Mega-AI Sundar do 1:1 Weekly check-ins with mini-AI Sundar?